Access a range of datasets and data tools to further your wildfire research.
Wildfire is an essential process connecting terrestrial systems to the atmosphere and climate. As vegetation burns, it releases smoke, carbon, and other materials into the atmosphere. These fires also release nutrients into the soil and are an integral part of ecological succession, plant germination, and soil enhancement. However, wildfires can also pose a hazard to human health and life. The 2022 Annual Report by the National Interagency Coordination Center (NICC) notes that "a total of 2,717 structures were reported destroyed by wildfires in 2022, including 1,261 residences, 1,294 minor structures, and 91 commercial/mixed residential structures."
The number, severity, and overall size of wildfires has increased, according to the U.S. Department of Agriculture, through contributing factors including extended drought, the build-up of fuels, past fire management strategies, invasive species targeting specific tree species, and the spread of residential communities into formerly natural areas. In 2022, 68,988 wildfires were reported across the U.S. that consumed 7,577,183 acres, according to the NICC report.
Wildfires Near Real-time Data
NASA's Fire Information for Resource Management System (FIRMS) distributes global near real-time (NRT) active fire data from Earth-observing satellites, and provides enhanced interactive tools to help predict, prevent, and manage wildfires. NASA’s satellites also provide other data useful to the study of wildfires, such as forest cover, temperatures, lightning, burn scars, and air quality.
Learn more about near real-time fire data provided by FIRMS and NASA's Land, Atmosphere Near real-time Capability for Earth observation (LANCE).
Learn How to Use Wildfires Data
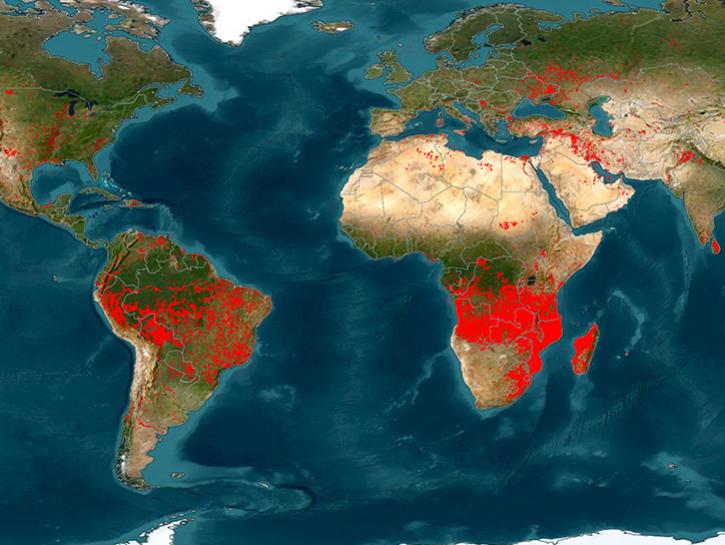
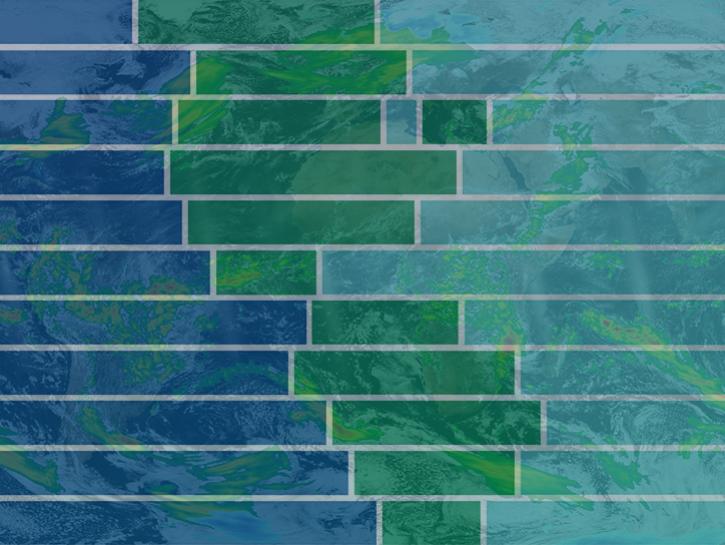
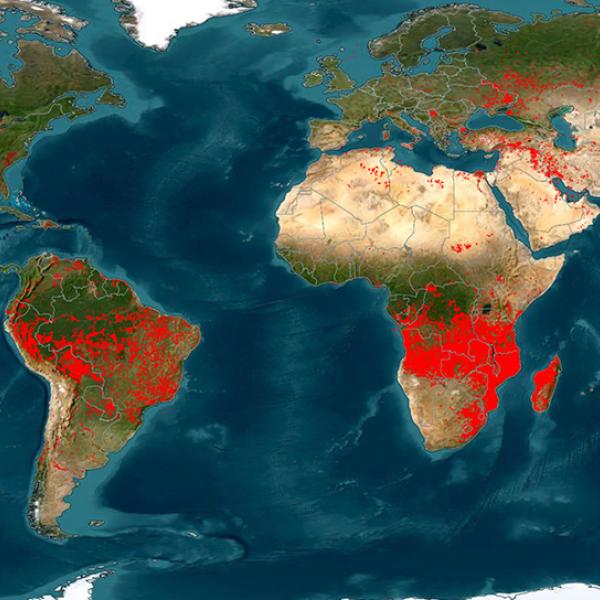
Join Our Community of NASA Data Users
While NASA data are openly available without restriction, an Earthdata Login is required to download data and to use some tools with full functionality.
Learn About the Benefits of Earthdata LoginFrequently Asked Questions
Earthdata Forum
Our online forum provides a space for users to browse thousands of FAQs about research needs, data, and data applications. You can also submit new questions for our experts to answer.
Submit Questions to Earthdata Forumand View Expert Responses