Access a range of datasets and data tools to further your research into diseases and epidemics.
The World Health Organization (WHO) notes that disease outbreaks are often associated with environmental conditions. Changes in water and air quality, for example, can affect disease transmission. Environmental factors such as temperature and precipitation can also impact disease transmission by changing the habitat suitability for organisms that can transmit infectious pathogens, like mosquitoes, ticks, and rodents.
Changes in temperature and humidity also play an important role in seasonality trends for diseases. As observed from the COVID-19 pandemic, disease outbreaks can lead to environmental changes due to altered human behavior, such as decreased vehicle use and stay-at-home measures leading to reductions in greenhouse gases.
Sensors aboard Earth observing satellites cannot detect the spread of diseases from space. However, they provide long-term data records that help address, inform, and monitor many of the factors mentioned above, including air and water quality, habitat suitability, seasonality, and changes in Earth's environment due to changes in human behavior. NASA's relevant data products include hourly surface air temperatures, rainfall estimates, and nighttime lights.
Learn How to Use Diseases/Epidemics Data
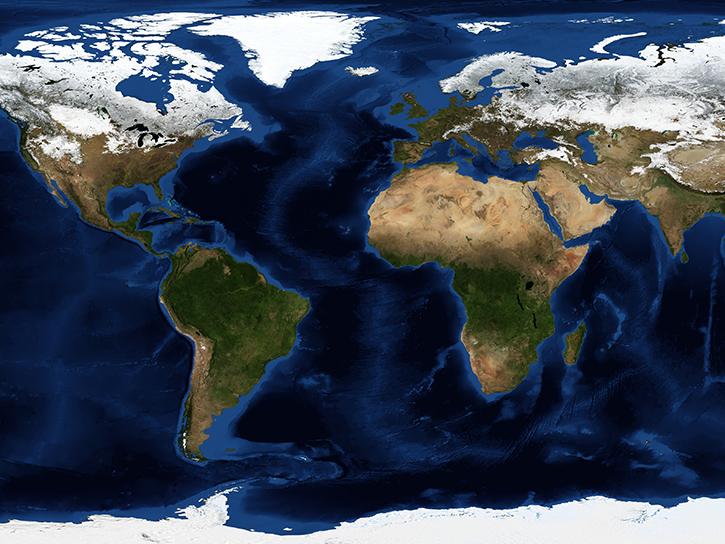
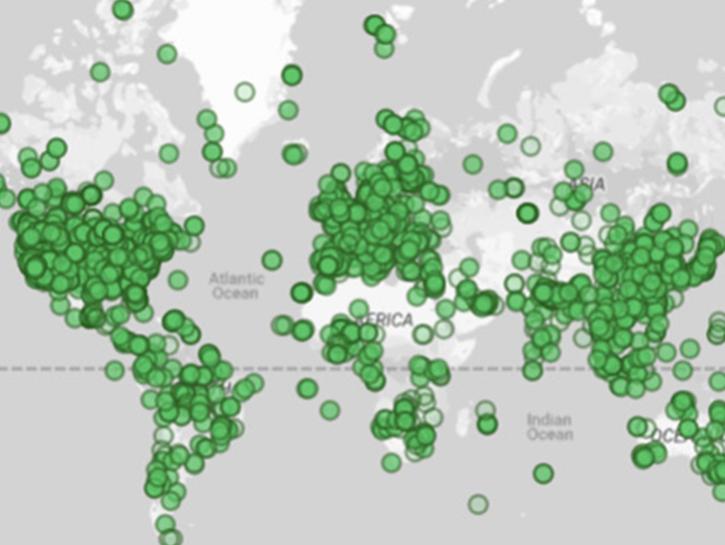

Join Our Community of NASA Data Users
While NASA data are openly available without restriction, an Earthdata Login is required to download data and to use some tools with full functionality.
Learn About the Benefits of Earthdata LoginFrequently Asked Questions
Earthdata Forum
Our online forum provides a space for users to browse thousands of FAQs about research needs, data, and data applications. You can also submit new questions for our experts to answer.
Submit Questions to Earthdata Forumand View Expert Responses