Access a range of datasets and data tools to further your research.
The ideas of people, planet, and prosperity underpin the 2030 Agenda for Sustainable Development, an international plan signed by all United Nations (U.N.) member states in 2015. This plan outlines 17 Sustainable Development Goals (SDGs), with 169 underlying targets. The plan was developed by a U.N. working group comprised of representatives from 70 countries, who gathered information and engaged with global communities to determine what the SDGs should include.
The vision of the SDG framework encourages every country to assume responsibility for planning and providing better outcomes for future generations, leaving no one behind. Participating countries are tasked with developing sustainable solutions for each goal by the year 2030; progress toward meeting these goals is measured by 230 different indicators. The plan promotes the triple bottom line concept of sustainability: every solution must consider and account for social justice, a healthy environment, and economic viability.
NASA Earth observation (EO) data are playing a key role in assessing progress toward meeting the SDGs. EO data inform our daily lives, providing information that enhances our understanding of the interconnectedness of people, the planet, and prosperity. EO data are used in tracking variability of life, assessing the impact of disasters, managing natural resources, observing land use changes, and mitigating climate change. The Group on Earth Observations (GEO), of which NASA is a partner, has developed a coordinated and consistent set of observations that can be used in assessing many of the SDGs.
Learn How to Use Sustainable Development Data
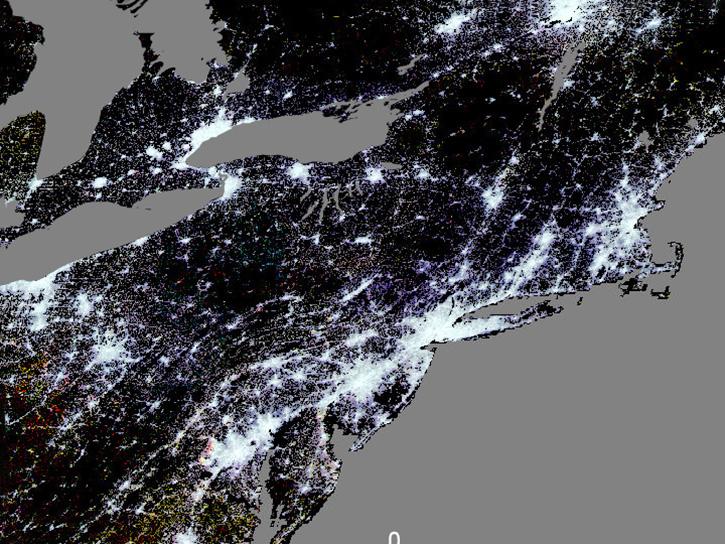
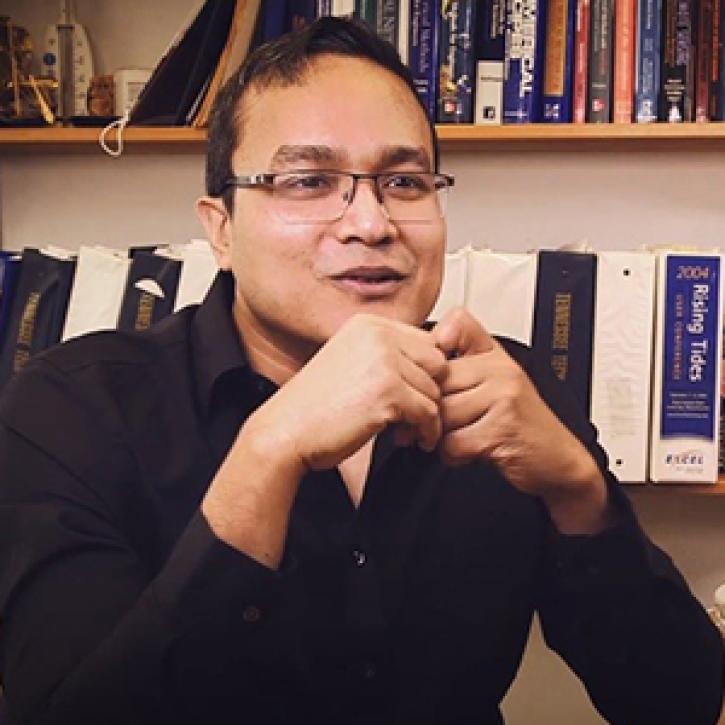
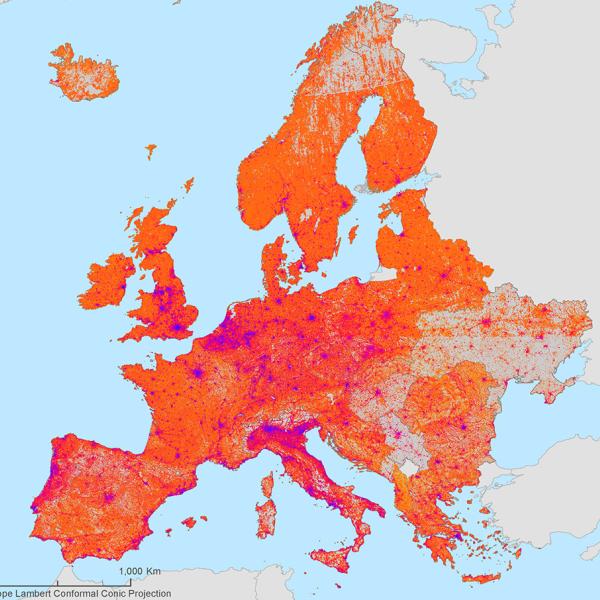
Join Our Community of NASA Data Users
While NASA data are openly available without restriction, an Earthdata Login is required to download data and to use some tools with full functionality.
Learn About the Benefits of Earthdata LoginFrequently Asked Questions
Earthdata Forum
Our online forum provides a space for users to browse thousands of FAQs about research needs, data, and data applications. You can also submit new questions for our experts to answer.
Submit Questions to Earthdata Forumand View Expert Responses